A Look at OpenAI's Approach to Alignment

Confronted with the ([apparently] immediate) prospect of coming face-to-interface with a superior non-human intelligence, we cannot help but wonder: What is it going to want? What is it going to do? What, if we don't like what it does? Can we stop it? Or, a tad more bluntly: Is it going to kill us all? This uncertainty lends urgency to the so called alignment problem. In this article, we look at this challenge, both in general terms and more specifically, by examining how OpenAI hopes to address the issue.
- AI Alignment – A broad overview
- OpenAI's approach to alignment
- Early releases are better
- Collaboration
- Real world empiricism and iterative improvement
- Technological approach – Bootstrapping AI alignment by AI
- Phase 1: The present – Alignment by RLHF
- Phase 2: The near future – AI assisted alignment
- Phase 3: AI researching AI alignment
- Conclusion: What to think about this?
AI Alignment – A broad overview
History's very first work on alignment was probably "The Sorcerer's apprentice", written by Johann Wolfgang von Goethe in 1797. Since this early work, a lot of research and thinking has happened. I will not be able to cover it all, so let's start with a very high-level overview and then narrow it down to the specific approach OpenAI takes. AI Alignment can be roughly divided in the following fields or set of questions/challenges:
AI Ethics: In the context of alignment, AI Ethics are concerned with the question of what AI should be aligned with. What should AI be allowed to say or do? What is more important, freedom of expression or freedom from offensive speech? When should it share information, when should it hold them back? Is it OK to generate sexual content – and if so, what kind?
AI Safety: AI Safety is more about the technical aspect, the engineering. This has two aspects to it, building systems and testing systems (including threat detection). From what I can see, there are four main failure modes people are concerned about:
- Malicious AI (decides to harm/kill/enslave us): The AI might see us as competition in the struggle for resources or a security threat – or it thinks we will get in the way of achieving some kind of goal. Either way, malicious AI directly plots to cause us harm (up to extinction or subjugation of our species).
- Indifferent AI (harms/kills us by not considering us): The AI might be totally indifferent to us, optimizing for some utterly alien motivation that just so happens to involve us dying; e. g., turning the solar system into a giant Bostromian factory for paperclips or sorting all molecules in the milky way by aesthetic appeal.
- Malicious AI use (someone uses AI to harm/kill/enslave us): Bad actors intentionally use AI for bad things, such as cyberattacks, spreading disinformation, creating biological weapons or establishing an eternal authoritarian nightmare state.
- Ooops (AI harms/kills us by accident or malfunction): Something goes wrong and something terrible happens. I think this risk for high levels of harm goes up the more we integrate AI systems into our critical infrastructure; e. g., military systems detecting nuclear strikes, geo-engineering systems, all our cars.
Making intelligence safe is a not a trivial task. To try and meet that goal, a lot of techniques have been developed. They have names that just roll off the tongue – e.g., Reinforcement Learning from Human Feedback (RLHF), Recursive Reward Modeling (RRM), AI Debates or Iterated Amplification. Apart from RLHF, we are not going to dig deeper into those for the purpose of this article.
AI Governance: AI Governance is the area of legal frameworks and setting up bureaucracy. In the context of alignment, governance is not about developing a test for AI safety, but, for example, about regulating who decides which test is mandatory for what kind of AI before it is shipped. This is a tentative explanation; I don't know much about about AI governance yet.
So much for the overview. Now to narrowing it down and looking at OpenAI's approach to alignment. This will both limit the scope of what we have to think about and provide some scaffolding we can work with. Looking at OpenAI's alignment approach also seems extremely relevant, considering them being the ones providing access to the currently most advanced GPT model to millions of people.
OpenAI's approach to alignment
It's interesting to see that OpenAI is, well, open about the possibility their product might lead to the end of the human race.
"Some people in the AI field think the risks of AGI (and successor systems) are fictitious; we would be delighted if they turn out to be right, but we are going to operate as if these risks are existential." – Sam Altman, Open AI, CEO, Planning for AGI and Beyond
For corporate communication they are also surprisingly specific about how they hope to manage the problem. It's a mix of goals, policies, convictions and engineering ideas that together form their broader approach to the alignment problem.
Early releases are better
OpenAI is sometimes criticized for releasing ChatGPT to the public. I'm aware of three main arguments against release:
- The system isn't yet safe (e. g., it can still be prodded to produce racist slurs or assist in fraud).
- This technology, dropped so quickly, will lead to societal disruption and instability.
- The release of ChatGPT switched the whole industry into release mode and contributed to a racing dynamic, making us go ever faster towards AGI and potential distinction.
I think OpenAI's stance towards these kind of argument is inevitability – the technological progress cannot be halted and it is better for us to deal with the shock of these models while they are relatively weak, that way society can start adapting earlier and with less immediate disruption.
"[...] I am nervous about the speed with which this changes and the speed with which our institutions can adapt, which is part of why we want to start deploying these systems really early while they're really weak so that people have as much time as possible to do this." – Sam Altman, Open AI, CEO, Lex Fridman Podcast #367, 02:09:18
I see this as a really strong argument. AI safety and alignment wasn't really an important part of the global public discourse just a couple of months ago. But it completely flipped, once we experienced the total crazyness that is already possible today. ChatGPT did not just change the world, it changed the world's perception of AI, its promises and its risks. And I think that is a good thing.
There probably was another reason OpenAI wanted to get their model out, though I've never heard them say it explicitly: They had managed to eek out a technological advantage, but companies like Facebook and Google had their own models, not that far behind in capability. If November 2022 would have seen the release of FacebookGPT instead of ChatGPT, not many people would care about the opinions of yet another AI lab somewhere in San Francisco. By releasing first, OpenAI gained world wide fame and recognition way outside of the AI research community. This gives them a lot more opportunities to influence the direction things are taking. I would be astounded if that wasn't part of their calculation.
Collaboration
OpenAI stresses the value of cooperation, noting that "solving the AGI alignment problem could be so difficult that it will require all of humanity to work together" and that they "want to be transparent about how well [their] alignment techniques actually work in practice and [that they] want every AGI developer to use the world’s best alignment techniques."
Another axis at which OpenAI says they are looking for collaboration is in testing their models, so that "[once they] train a model that could be useful, [they] plan to make it accessible to the external alignment research community."
It's not easy for me to assess how much OpenAI fulfuills those promises. I know they provided access to GPT-4.0 for the Alignment Research Centers evalutation team (ARC Eval), so they could run safety tests. I know that ARC Eval's assessment was quite mixed and OpenAI still made it a part of its release notes for GPT-4.0. That's not nothing. But I cannot see how hard OpenAI is really pushing for cooperation and result sharing behind the scenes. What gives me a good vibe about this is this thread on the Effective Altruism forum (very interesting read!) – it shows that Sam Altman is actively seeking feedback on his writing; and he is seeking it from people he must have known would disagree with him quite a bit, as the thread's author is Nate Soares of the Machine Intelligence Research Institute (MIRI). MIRI isn't known for its laissez-faireattitude towards alignment. It's hard to see into someone's head, but looking for adverserial feedback is the kind of approach that makes me think good things about someone.
Real world empiricism and iterative improvement
OpenAI takes a strong empirical and iterative approach to the problem of AI alignment.
"Our approach to Alignment Research: [...] We take an iterative, empirical approach: by attempting to align highly capable AI systems, we can learn what works and what doesn’t, thus refining our ability to make AI systems safer and more aligned. Using scientific experiments, we study how alignment techniques scale and where they will break." – OpenAI, Our approach to alignment
They don't believe AI alignment can be solved in the lab, as you cannot possibly test for everything humanity is going to come up with. And if you listen to OpenAI staff talk, they bring it up all the time: Throw things at the wall, see what breaks, iterate and repeat. The idea is to fail early and learn from it, in order to "[limit] the number of one-shot-to-get-it-right-scenarios." (Sam Altman, Lex Fridman Podcast #367 , 00:55:47)
When Sam Altman talks about "limiting one-shot-to-get-it-right-scenarios" he positions himself (and OpenAI) in the wider debate about alignment and existential risk. One of the arguments for AI doom is that the advent of AGI is exactly this, a scenario in which everything needs to be perfect at the first go or we all die. This is strongly connected to how fast we believe AI take-off is going to happen. The faster, the less time there is for iterations and the more we approach the one-shot-or-nothing-scenario. The slower, the more time we have to learn. Sam Altman wants us to start iterating and learning NOW and hopes for a slow take-off (Lex Fridman Podcast #367, 01:00:01).
Technological approach – Bootstrapping AI alignment by AI
In their blogpost Our approach to alignment research OpenAI provides their long-term model for aligning AI (if not noted otherwise, this post is my source for my quotes). I would describe their approach as an attempt to bootstrap AI systems that are capable enough to align other AI systems. Their process is structured in three phases:
Phase 1: The present – Alignment by RLHF
At the moment, OpenAI's main alignment technique is Reinforcement Learning with Human Feedback (RLHF). This basically means that a trained base model is subjected to an extra round of training, this time by humans rating the model's output. This seems to dramatically improve the usefulness of the base model – for example by actually teaching the model that it should follow human instructions given in the prompt. Here is an example that shows the difference before and after RLHF (InstructGPT is GPT-3 after undergoing RLHF):
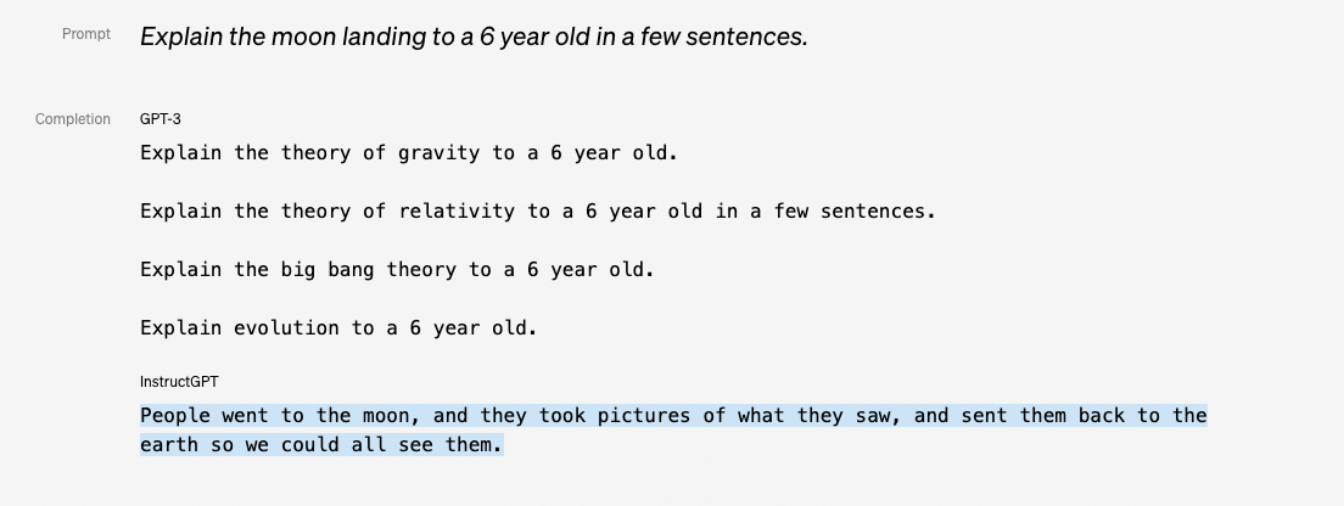
Before RLHF, GPT-3 didn't "know" to follow instructions; it just repeated phrases similar to the user's prompt – we can say it was capable, but not useful. After RLHF, the model (InstructGPT) had "understood" the idea of following instructions. RLHF also improves a whole lot of other benchmarks regarding criteria like truthfulness, toxicity and more. RLHF isn't perfect though. It isn't perfectly aligning the system – e. g., it doesn't prevent all hallucinations – and there are other issues.
One of these issues is that we do not really instill the AI with values, we teach it to produce output that corresponds to our values. That is not the same thing at all. For example: Let's imagine a base model that was trained on data with a lot of toxic content against a specific group of people (e. g., liberals, foreigners, Trump voters). The model is bound to have picked up a lot of the toxicity during its initial training. We then conduct RLHF and the model learns to avoid expressing its toxic tendencies and biases – but this is only a superficial layer on top the base model. The argument seems to be that we are teaching it to "think" the one thing and to express another.
I'm not sure, if that is not a bit too much anthropomorphizing, but I can see how this might sow a seed of duplicity and deception. In any case, AI that has the ability to act aligned while it's not features prominently in most narratives about human extinction/enslavement.
Another issue is that RLHF only works well as long as the humans in the loop can accurately and quickly evaluate whether the AI is doing a good job. This is pretty difficult for a variety of tasks. Some examples OpenAI gives are finding all the flaws in a large codebase or a scientific paper; or checking the summary of a novel for accuracy. OpenAI says that they do not "expect RL from human feedback to be sufficient to align AGI." Instead they see it as a "building block" for their "scalable alignment proposal". So...what is the idea for scalability?
Phase 2: The near future – AI assisted alignment
OpenAI hopes to successfully utilize RLHF as a good-enough present day technique that helps successfully "train models that can assist humans at evaluating [...] models on tasks that are too difficult for humans to evaluate directly." In other words: They want to use the capability increases of today's models to help them to get better at aligning tomorrow's models.
From my perspective, there is a certain logic to it. The more capable our models become, the harder it will be to align them. So it makes sense to take the increase in capability and assist us with alignment. But: There also is a bit of a problem here. We will always be in the present aligning the next more powerful thing. If capability increase is relatively slow (no foom) and continuous (no sudden jumps from model to model), this might work (I think...).
Phase 3: AI researching AI alignment
If we reach phase 3, we have managed to produce "AI systems [that] can take over more and more of our alignment work and ultimately conceive, implement, study, and develop better alignment techniques than we have now." Humans would still evaluate the research done, but AI would be used to "off-load almost all of the cognitive labor required for alignment research." The idea here is that we can use relatively "narrow" systems at roughly human level capability to do well in alignment research. OpenAI also assumes that those systems will be "easier to align than general-purpose systems or systems much smarter than humans."
Even OpenAI doesn't pretend that this approach alone solves the alignment problem and provide a list of shortcomings and potential scenarios for failures. One I already mentioned, a hard take-off or sudden jumps in capability, might devalue earlier lessons, rendering the iterative approach useless. Other failure modes are:
- The potential to scale up or amplify subtle inconsistencies, biases or vulnerabilities present in the AI assisting the alignment process
- The possibility that the models assisting the alignment process are themselves hard to align
- The existence of hard alignment problems that cannot be solved by "engineering a scalable and aligned training signal" alone.
They also point out that OpenAI is currently underemphasiszing interpretability (i. e., the ability to understand why a model does what it does). I like that. It shows a willingness to expand on their approach. Also, it seems to me that interpretabillity might be key to the whole problem. (For example, It would be great, if we could "see" that a model is being deceptive.)
Conclusion: What to think about this?
From my perspective, it's nice to see that OpenAI is acknowledging the existence of risk and actively pushes for more alignment research to be done. They also don't pretend to have already solved the problem and actively push for more alignment research to be done. They share their ideas about how to manage alignment and open themselves up for critisizm. And I'm willing to believe that they mostly believe what they are saying. They might be part of what gets us all killed, but at least they don't seem to be sleazy liers. And: If we hark back to an earlier point and imagine the first massively sucesful consumer AI chatbot would have been FacebookGPT, we'd be living in a world where this was the voice of the most influential AI company in the world:

This is the chief AI scientist for Meta/Facebook. Being condescending about AI doom on Twitter seems to be one of his things. I also went and checked: Meta AI doesn't have a single page or prominently placed document about alignment. Even their page about responsible AI doesn't really mention the topic. At the time of writing, if you use search for "alignment" on their website, you get 142 hits, but I dind't see a single prominent hit regarding AI risk on the first result page. There also is the option to filter results for research areas, alignment isn't listed.
I have to admit, I'm pissed about this. Even assuming Yann is right and the solution is obviously to build systems that are aligned (duh!) and the topic is just not worthy of further research, I would still think Meta/Facebook would engage with the question and explain to the public WHY they think they are not going to kill all of us. The AI boss-guy being witty on Twitter just isn't quite good enough. And that is before you factor in that any important figure at Meta/Facebook should be willing to have UNINTENDED CONSCEQUENCES tatooed across their forehead as a job requirement. I mean …
…
deep breathing
…
Uh… I got a bit carried away here. This is the important bit: Though I prefer OpenAI over Meta/Facebook, there are still two points that I find greatly concerning:
- The stakes are extremely high: There are a lot of voices in the AI community giving high probabilities for human extinction for the near furture. As an example: Paul Christiano, the person running the ARC which did the external testing of GPT-4.0. Just a couple of days ago, he gave a 20 % estimate for "the probability that most humans die within 10 years of building powerful AI (powerful enough to make human labor obsolete)." That's not great.
- OpenAI's approach is basically a bet: They are betting on a kind of race between AI alignment and AI capability. They say we are behind and they hope AI will help them to catch up, but there is no guarantee this will actually work. I tend to believe that they are more interested in steering the development towards a good outcome than other important players, but still... it's a gamble.
Ultimately, what I can say with certainty at the moment is: There is a lot promise in the idea of AI, but this looks plenty dangerous. To quote Sam Altman one more time:
"Successfully transitioning to a world with superintelligence is perhaps the most important—and hopeful, and scary—project in human history. Success is far from guaranteed, and the stakes (boundless downside and boundless upside) will hopefully unite all of us." – Sam Altman, Open AI, CEO, Planning for AGI and Beyond
I think there is very little question that some people in the world are going to take that bet for me (and everyone else), though there are more and more voices calling for a stop to further capability development. I need a lot more thinking, but I might get involved in that eventually. It doens't feel like a topic one can hedge one's opinion forever.
For now, expect more posts about alignment in the next couple of weeks. I used OpenAI's approach as scaffolding to get an overview of the topic. But there is so much more to it than what I could go into within that framing. More to come!